Why You Should be Building a Digital Twin
- Paul
- Jul 30, 2021
- 5 min read
An Important Emerging Digitisation Technology
What are Digital Twins?
Digital Twins are pairings of real world objects or systems, and computer models, via bidirectional flows of information - Data is not only streamed from the asset, but predictions that enable decision-making are returned in kind (in contrast to classic simulations, where the information flow is unidirectional).
In this article we'll look at some of the ways they can be applied to business and engineering problems, driving innovation and reducing ownership costs for a wide range of market segments.
Modern Digital Twins span the full product lifecycle - Think connected cars, digitised aeroengines, and smart factories, with all the functions, readings and outputs represented in a digital format and updated via connected sensors. Coined as a term by NASA in 2010, the Digital Twin was conceptually developed by Michael Grieves of the Florida Institute of Technology, and presented at a Society of Manufacturing Engineers conference back in 2002.

Fundamentally, the Digital Twin is a logical construct - It's a grouping of data and information to form a picture of a physical asset, with information flows between the real and digital versions. There are three distinct elements:
The physical object
The digital representation of the object
Connections between the two, through sensors, the internet and people
How does Machine Learning fit in?
Machine learning can be used alongside Digital Twins to analyse the data being returned by the physical version, and indeed to build predictive models from the digital version. This is a rapidly growing application area for machine learning technologies, and one that will be important in taking the predictive capability of digital twins to an enhanced level.
Digital Twins Create New Ways of Solving Business Problems
Digital Twins are about understanding (through clear visualisation) current product performance, making predictions about how the product will perform in the future, and using these predictions to make decisions (eg. when do we schedule maintenance?). This can generate opportunities for innovation and optimisation, reducing costs or improving quality and reliability.
In practice, Digital Twins can be differentiated into three distinct categories: Prototypes, Instances and Aggregates, with subtle variations in the structure and objectives of the system.
The Prototype Twin (or DTP)
The DTP exists before the physical product does, and it consists of the design, analysis and modelling data used to define the asset. The DTP serves as an umbrella over system, component and control models, linking them together and circulating feedback such that design and development decisions can be taken with reduced prototyping.
Early mistakes in the product development lifecycle result in very expensive redesigns and delayed launches. DTPs allow early intervention and avoid investment in expensive prototypes and physical tests.
DTPs are particularly useful for technology innovation in environments of extreme uncertainty - Where there are major unknowns about the user/customer, about the operating environment, and about the capabilities of the technology.
Example: Autonomous Vehicle Development
Autonomous vehicles are an excellent example of DTP application, as one of the major hurdles in developing control systems that can react to unpredictable events is in training the system without incurring the massive expense of physically testing in such a diverse environment.
Control simulations can be connected to vehicle and driving environment simulations to generate millions of training scenarios, including rare and unpredictable events, to both train and assess the performance of the controller, and accelerate development.
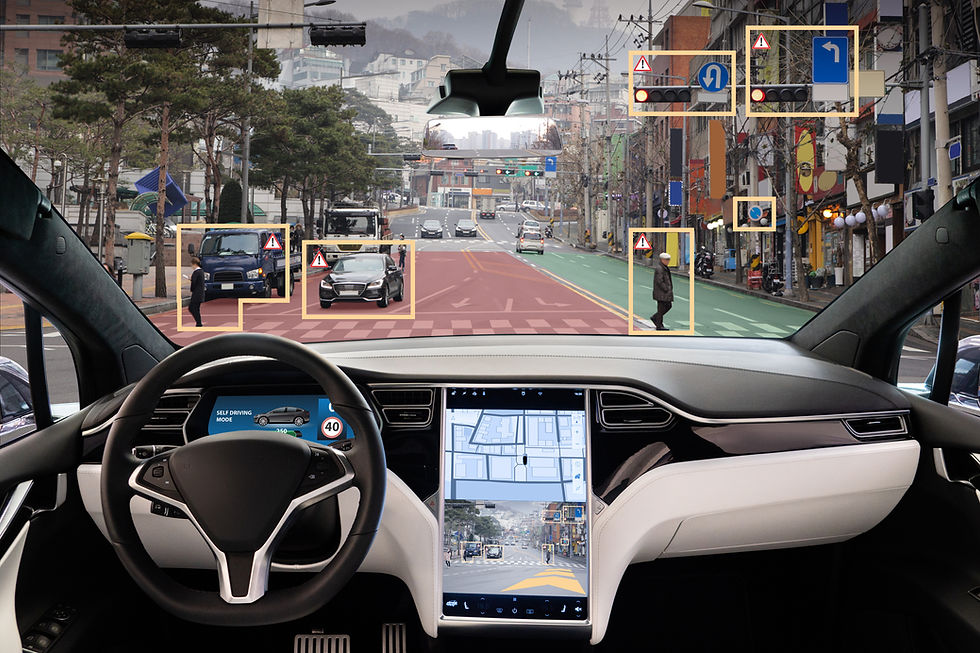
The Instance Twin (or DTI)
The DTI is the 'instance' Twin of each unit of an asset design, following it from manufacture into operation, maintenance and on to end of life. Each physical instance has an equivalent Digital Twin, which receives instance data and makes predictions around performance and degradation - These predictions can be used to make decisions around asset operation, servicing and maintenance.
The Twin can be expanded to include the digital histories of the parts used in their construction, provisioned by connectivity into supplier manufacturing and quality data stored in the cloud. This can help with accelerated quality control if an issue is identified with a particular production batch.
Instance Twin predictions are particularly useful for optimising asset maintenance, increasing up-time and significantly reducing reactive maintenance, which is vital for reducing operating costs.
Example: Gas Turbine Condition Monitoring
A number of gas turbine manufacturers (including Rolls Royce) now have the capability to remotely monitor individual stationary and aero engines for anomalous behaviour. Real engines stream a multitude of operational data via connected sensors, and this data is fed into models to report on performance and make predictions about when maintenance interventions may be needed.
Condition monitoring improves the ROI of the base engine by shifting the maintenance regime from reactive/expensive to planned/cost-optimised, maximising asset usage without compromising on performance or safety.
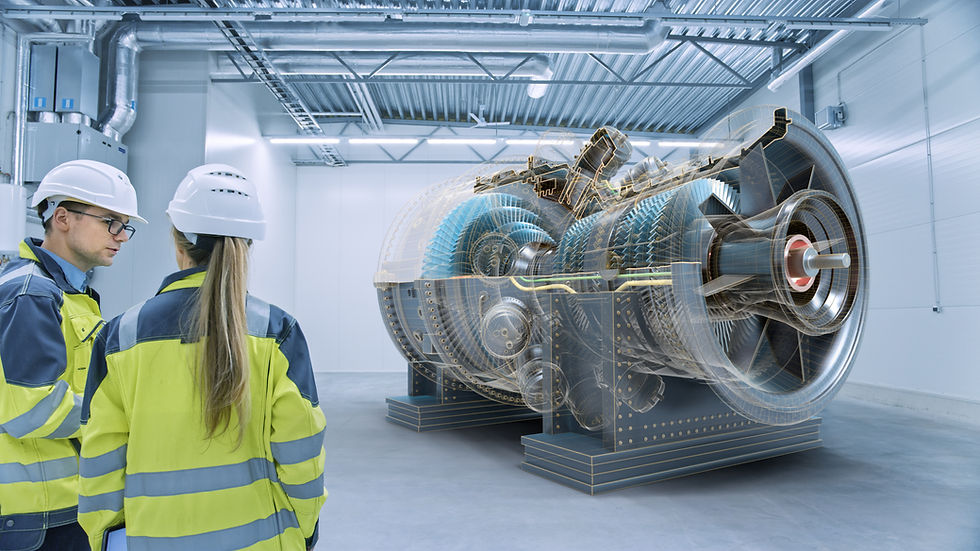
The Aggregate Twin (or DTA)
The DTA is the aggregate of instance Twins, forming a high-level picture of fleet or system performance and behaviour. Data flows in Aggregate Twins can be used for understanding the physical system in a general sense, for enhanced prognostics and for developing new product ideas and applications.
One way to think of Aggregate Twins is that they work with the meta-data generated by the instances - One example would be the summary statistics generated by a fleet of vehicles, ships or power plants; Another would be the data generated by the operations of an energy grid.
Aggregate Twins can be useful in understanding how a product in widespread deployment is performing and aging, as they generate comparisons between different instances operating under different conditions. This can be useful for optimising the operation of a given asset (improving ROI), and for identifying anomalous behaviour that might need an intervention (eg. this solar panel seems to be performing more poorly than others?).
Example: Maritime Fleet Operations
Maritime operations are an area of the transport system that is under considerable pressure to reduce carbon emissions. Aggregate Twins of maritime fleets can help by building fuel consumption models of shipping operations, which can then be used to identify vessel-route combinations with min. and max. efficiencies, particularly when weather and sea condition data is brought into the model.
These models can in turn be used to predict optimal vessel speed and navigation parameters, which can be returned via digital connection to the vessel and factored into operational decision-making. This type of aggregate modelling can also identify when a ship is consuming more fuel than expected, so that early maintenance can be planned and actioned at a cost effective point.
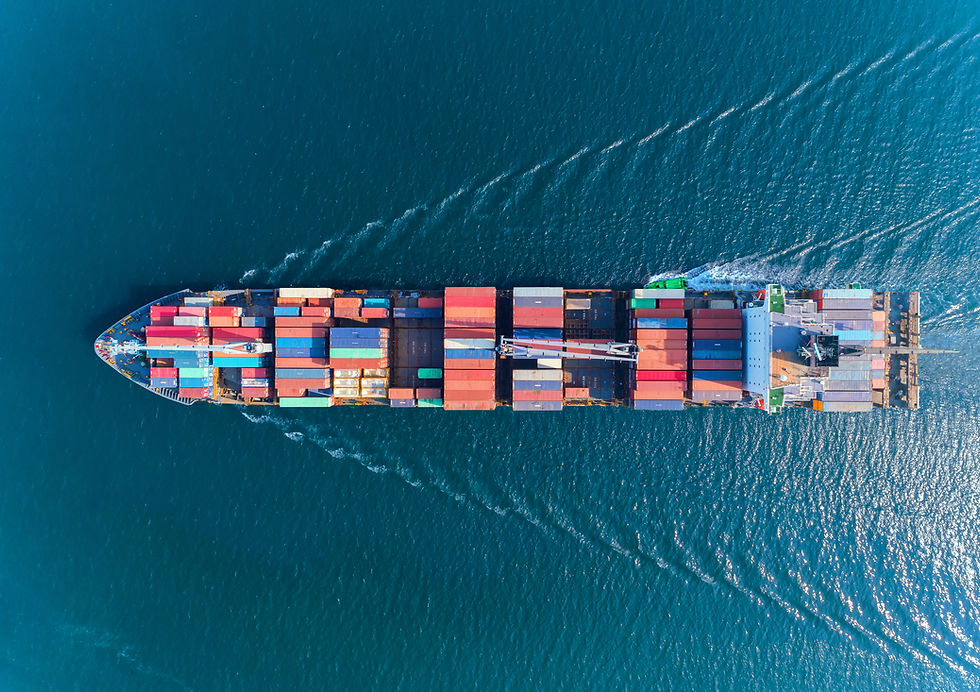
In Summary
Digital Twins are a technology that has been around conceptually for a number of years; However, over the last 5-10 years there has been a substantial acceleration in at least three dimensions: Number of applications, breadth of application and model fidelity. Much of this has been driven by increases in computing power and connectivity technologies like the cloud and IoT.
Although Digital Twins are currently seen as cool and fashionable, it is vitally important that they are designed and developed with a strong use case in mind - They have to be able to deliver improvements in product costs or quality, or to drive new innovation to be successful. The business case should be central to the goals of the twin.
Having said that, Digital Twins (when designed and implemented correctly) can provide fascinating and powerful insights into how assets and systems are working, which in turn drives better decision-making and improvements to the bottom line.
If you're thinking about developing a new or existing Digital Twin technology, talk to us today about how we can assist you.
I hope you enjoyed the article, and thanks for reading!
Paul
Comments